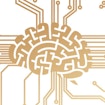
In this study, Elena Tenenbaum, Shafali Spurling Jeste and a team of researchers and clinicians, plan to capitalize on recent advances in remote administration of cognitive development tasks and computer vision software to pilot the Remote Infant Studies of Early Learning (RISE) Battery. This project represents a critical first step to increased understanding of early cognitive development in autism and has the potential to elucidate the foundational underpinnings of clinical heterogeneity in autism. This in turn will inform more targeted, developmentally relevant early intervention strategies for infants with atypical developmental trajectories.