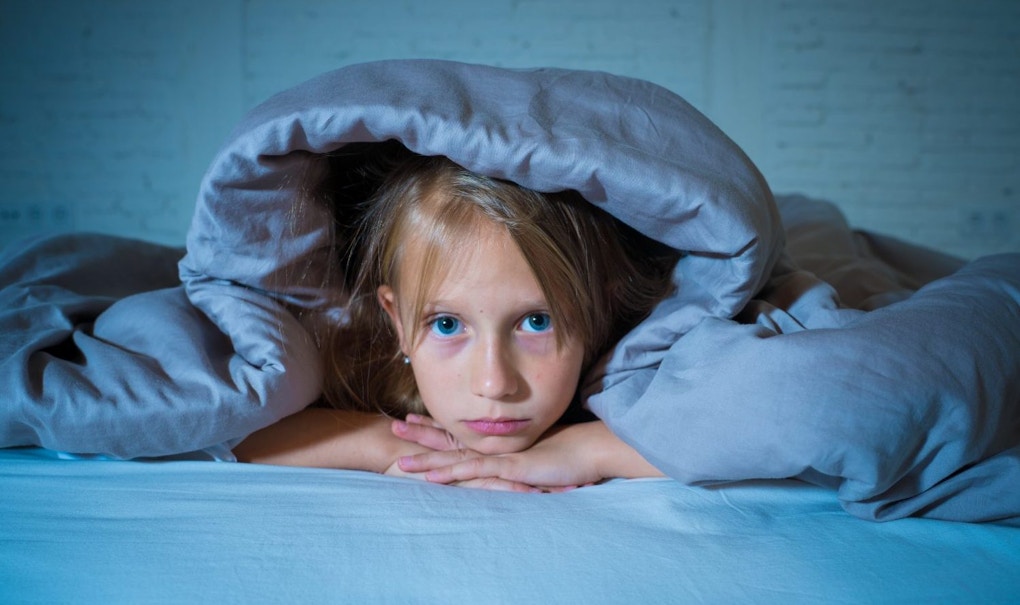
sam thomas/iStock
Sleep problems often accompany autism spectrum disorder (ASD), though poor sleep is not currently considered to be one of the core features of the condition. Yet sleep disturbances can worsen the severity of other autism traits and challenge family members and caretakers of people with autism. While the causal relationship between sleep disturbance and autism symptom severity remains unknown, finding ways to remedy sleep has the potential to make real improvements in the health and well-being of people with autism and their family members. To examine what is known about sleep in autism, and to brainstorm approaches to improving sleep, SFARI convened a two-day online workshop on June 25 and 28, 2021, that brought together diverse researchers, pharmaceutical companies and family organizations to investigate different aspects of sleep in ASD and related neuropsychiatric disorders.
Why is sleep important?
The importance of sleep was brought home by Geraldine Bliss, the mother of a son with Phelan–McDermid Syndrome (PMS) and co-founder of CureSHANK, which aims to accelerate treatments for PMS. PMS is caused by mutations to the SHANK3 gene, which encodes a scaffolding protein found at synapses. Bliss described how her son’s development gave way to speech delays, social difficulties, intellectual disability and eventually epilepsy. From the very beginning, however, sleep was challenging for him, and it remains so: as a young adult, he has difficulty falling asleep, wakes frequently and struggles to fall back asleep.
Bliss described how sleep influences her son’s propensity for seizures, his behavior, mood and daytime lethargy, and she emphasized that addressing sleep could greatly improve his quality of life. Parent surveys reveal that sleep problems in PMS are very common, and very severe, which leads many to experiment with diverse medications, including cannabidiol or chloral hydrate. “I have been dismayed that there is not more sleep research,” Bliss said.
As a sleep specialist and mother of two children with ASD, Beth Malow of Vanderbilt University Medical Center admitted to struggling with sleep issues in her own family. She emphasized that many sleep problems in ASD are treatable and that improving sleep can benefit quality of life and help the person engage in school or work. Malow argued for a holistic approach that considers biological reasons for disrupted sleep, such as changes in melatonin levels; medical reasons, such as obstructive sleep apnea, seizures, attention-deficit/hyperactivity disorder and medications used to treat these conditions that affect sleep; and behavioral reasons, such as caffeine intake, limited exercise and use of screens close to bedtime1. She also cautioned that while bringing a child in for a sleep study in the clinic can reveal what is disrupting sleep, such as seizures, apnea or leg movements, what this reveals about sleep itself can be limited given the artificiality of the environment and day-to-day variability in sleep patterns. Ideally, people would be monitored over weeks, and wearable technologies that can be used at home could be helpful for this.
Less sleep has been associated with more severe autism manifestations2 and upticks in irritability, hyperactivity and inattention3. Just as for anyone going without sleep, people with ASD and sleep problems will struggle to make progress in their therapies, and their parents and caregivers will struggle to support them. It is feasible to teach sleep improvement interventions to parents4 and community healthcare providers5. Malow noted that melatonin can shorten the time it takes to fall asleep, but overall studies of medicinal sleep aids in autism are small. Finally, she emphasized that the difficulty in falling asleep may be a sign of a circadian rhythm disturbance, rather than insomnia per se.
Measuring sleep
Sleep itself is a complex state, with distinctive stages involving different patterns of brain activity and physiological changes. The sleep architecture also changes across development, with REM sleep reduction at an early age followed by slow-wave sleep change as children age.
On behalf of Ruth O’Hara, Makoto Kawai of Stanford University described how polysomnography (PSG), the gold standard for sleep measurement and analysis, can be used successfully for people with autism. The technique is not necessarily well tolerated, however, because it involves sleeping with multiple sensors attached to the body: EEG leads to detect brain waves, electrooculograms for eye movements, electromyograms for muscle tone, electrocardiogram for heart rhythms and airflow sensors for the nose and mouth. Ambulatory PSG set-ups that work in the home are available, but even these require a prolonged desensitization period to allow a person with ASD to adapt6. Preliminary results from Kawai and O’Hara’s work suggest differences in slow wave sleep in ASD compared to people without ASD.
Sleep may both influence and reflect brain development. In the latter case, changes in sleep patterns may signal the emergence of atypical brain development before other symptoms emerge. For example, children with ASD show less REM and more non-REM than age-matched typically developing children, suggesting changes in neural organization7. To discern links between sleep and neurodevelopment, a prospective longitudinal study called the Electrophysiologic Sleep Phenotyping (ESP) Project has been set up as part of the Sleep and Neurodevelopmental Consortium, which has been collecting clinical, genetic and now EEG data around sleep. Ashura Buckley of the National Institute of Mental Health (NIMH) described the study, which aims to clarify links between sleep patterns and neurodevelopment and behavior, clinical features in NDDs and predictions of their course. Standardized and widespread collection of this kind of data will hopefully become a norm in the near future. “We should be extensively documenting sleep in all of its complexity,” Buckley said.
This sleep data is deposited in a large sleep registry maintained by the National Sleep Research Resource (NSRR). The registry contains data from PSG, actigraphy and self-reports for over 30,000 people and provides a platform for analyzing and sharing data between researchers8. The new data from the Sleep and Neurodevelopmental Consortium adds a pediatric and neurodevelopment focus to this repository. Open source software such as LUNA is also available to aid analysis and could help distill the aspects of sleep that are important to monitor. These include frequency power, as well as bursts of oscillatory activity called spindles, which reflect thalamocortical circuit function. Much of the sleep data in these databases are at risk for ascertainment bias, as registrants are referred for a sleep study, largely to rule out sleep apnea. Buckley said that the ESP Project and the Sleep and Neurodevelopment Consortium are also collecting data from those showing typical development and could potentially incorporate data from actigraphy or other devices.
Slow waves and sleep spindles
Feeling refreshed after sleep not only requires getting enough sleep, but also the right kind — that is, the deep sleep characterized by slow oscillatory activity. Reto Huber of the University Children’s Hospital Zurich described how new technologies can help monitor both sleep quantity and quality: for example, measuring smartphone taps can be used to quantify sleep with improved accuracy and capture wakefulness when wrist-worn actigraphy does not9. In the lab, slow oscillatory activity in specific scalp locations differ between psychiatric conditions, and some symptoms correlate with these signals. To establish causal connections, however, requires manipulation experiments. Huber described his SleepLoop system, which monitors sleep EEGs through a headband and delivers a tone stimulus at a specific frequency to entrain brain rhythms without waking the sleeper or altering the amount of slow wave sleep. Acoustic stimulation can boost or diminish slow waves during sleep, which affects learning efficiency in healthy adults10, or alter coupling between spindles and slow waves important for memory consolidation during sleep11. This suggests that such a system could be used to boost the restorative power of sleep. An in-home version of SleepLoop has been developed to allow monitoring across multiple nights, and parents can be coached on its use in their children.
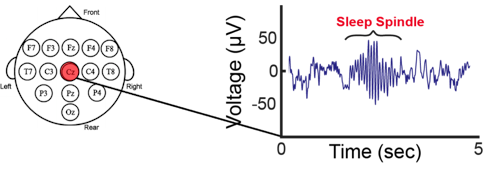
Sleep plays a critical role in memory consolidation, with sleep spindles identified as mediators of improvements in motor skill learning. Dara Manoach of Harvard Medical School described her work focused on people with schizophrenia, who exhibit fewer sleep spindles, which is related to their lack of sleep-related improvement in motor skill learning12 and IQ scores13. Paradoxically, treatment with eszopiclone increased sleep spindle density in people with schizophrenia but did not improve their memory consolidation14. This likely reflects that eszopiclone altered the timing of thalamic spindles relative to cortical slow oscillations and hippocampal ripples. It is the dialogue between these three non-REM sleep rhythms that transfers new memories from temporary representation in the hippocampus to longer-term storage in the cortex. Stimulation devices similar to SleepLoop may be able to increase spindles while preserving the precise timing of these three oscillations.
Manoach also shared preliminary data showing a mistiming of the coupling between spindles and slow oscillations in children with ASD, consistent with other evidence showing changes in interactions between the thalamus and cortex. Some autism risk genes encode channels found in the thalamic reticular nucleus, which generates sleep spindles and mediates thalamocortical interactions. She suggested that a genetic hit to a channel important in the thalamic reticular nucleus early in life may interfere with the establishment of thalamocortical circuitry, which in turn could set the stage for development of ASD.
Sleep endpoints
Sleep has also captured the interest of researchers at pharmaceutical companies because it might provide biomarkers to help monitor a drug’s effect. Sleep problems are common in neurological conditions, including NDDs, and could index disease progression or even worsen it. Sebastian Holst of Roche described his team’s study of sleep in Angelman Syndrome (AS), a rare neurodevelopmental condition marked by sleep disturbances such as short sleep, as part of its effort to develop medicines for AS. Parents report sleep as a key area for improvement in AS, which has prompted Roche to looking at sleep features that could be used as endpoints in clinical trials, as part of FREESIAS (Endpoint Enabling Study in AS). This study involves multiple home visits over the course of a year, and in the realm of sleep includes data from PSG, actigraphy devices, an under-the-mattress sleep mat to detect movement, as well as a mobile phone-based sleep diary and heart rate and respiration sensors. Holst noted that people with AS show unusual sleep-like EEG waveforms while awake, with high signal in the delta range between 0.5 and 4 Hz15. This means multiple methods will be needed to identify periods of sleep or wakefulness. Video monitoring could help but would need to be anonymized or outsourced to assure ethical use.
Although sleep concerns are common among parents of infants, some might provide cues in children who will later develop ASD. As part of the Infant Brain Imaging Study (IBIS), Annette Estes of the University of Washington and colleagues have analyzed parental reports of sleep among infant siblings of children with ASD, who carry a higher risk for the condition themselves. Sleep onset was more challenging at 6 and 12 months of age in those who later received an ASD diagnosis than in those who did not16. Sleep could compound behavioral issues, too: at age 4, sleep problems were associated with worse restricted and repetitive behaviors in those with ASD and with developmental delays as compared to typically developing children17. Whether these sleep problems predict the developmental course in ASD remains unclear, and to resolve this requires moving from parental reports to more objective measures of sleep.
Animal studies may help researchers find the most informative sleep measures. Lucia Peixoto of Washington State University outlined similarities in sleep between ASD and mouse models, such as those engineered to lack the SHANK3 gene, which is mutated in PMS. Adult mice missing SHANK3 show a longer latency to sleep compared to control mice, which resembles the difficulty in falling asleep commonly reported in people with PMS. Following sleep pattern development can also be revealing: a longitudinal study of sleep showed that wild-type mice undergo significant restructuring of their sleep between postnatal days 23 and 29, whereas mice missing SHANK3 seem to retain an immature state of sleep18. The work suggests an important sleep transition between infancy and toddler age. To study sleep in people in naturalistic environments, Peixoto and colleagues are validating the use of non-contact motion biosensors (“nearables”) that can be used in the home to monitor sleep. These “nearables” accurately measure sleep time, sleep onset and sleep staging19, while avoiding the potential sensory sensitivities of “wearables.”
Sleep studies in the home
Maja Bucan of the University of Pennsylvania argued for incorporating sleep phenotypes in genetic studies with wrist-worn devices. She and colleagues have developed a cohort of people with ASD without intellectual disability, as well as their family members. Designed as a genetic study, the cohort has several domains of phenotypic information collected, including sleep data captured by an actimetry wristwatch-like device, which not only captures motion, but also temperature and ambient light in the home (GENEActiv). A pilot study of nearly 400 people has found some significant differences in sleep measures in those with ASD and their unaffected relatives, as well as some correlations between sleep parameters and measures of social difficulties and executive function. The actimetry data, analyzed by ChronoSapiens software, can also detect cycles of locomotor inactivity during sleep (LIDS) that correspond to cycles of REM and non-REM sleep. Eventually, sleep phenotypes will be integrated with genomic analysis of known circadian genes or genetic loci associated with autism.
Approaches to studying sleep in other conditions, such as dementia, can inform methods for ASD, said Derk-Jan Dijk of the University of Surrey. To begin to validate sleep measurement methods in dementia, Dijk and colleagues have tested multiple technologies to monitor sleep in the home in cognitively-healthy older people. This showed reasonable agreement between data collected in the home from an EEG headband to EEG signals collected in the lab using gold-standard PSG methodology. In contrast, data from a mattress sensor did not correlate with PSG sleep stage measures, yet still provided useful information about when the person was in bed and interruptions of the sleep period. Other approaches focus on combining ambient light information with mathematical models of the circadian system to design individualized light interventions for circadian disruptions, or use of transcriptome biomarkers that can index circadian phase20 or sleep deprivation21 from only a few blood samples.
Ilan Dinstein of Ben-Gurion University of the Negev described the National Autism Database of Israel, which is a longitudinal collection of genotype and phenotype data22. Sleep measures are among the many collected phenotypes, which include parent report and lab-based PSG data. Sleep sensors that can be used at home may bridge the gap between parent reports and PSG, as well as offer long-term data that can characterize circadian rhythms and sleep homeostasis. For this, Dinstein’s team has been experimenting with the Fitbit activity monitor. In college students without ASD, they find some agreement between the Fitbit and PSG on sleep duration and epochs of slow wave sleep or REM. Preliminary results in children with ASD find that most will tolerate a Fitbit taped to their arm for five consecutive nights and that these provide data about night awakening, sleep onset and wakeup times that were mostly matched to parent reports. Incorporating sensors of heart rate, oxygen saturation, breathing and ambient light to activity could provide a more refined and personalized picture of sleep.
A downside of the Fitbit is that the operational algorithms are secret, which prevents researchers from obtaining its raw data. These algorithms may also change with new models, which give researchers even less control. Other devices may offer alternatives, but any device will have to be validated against a standard measure to get a sense of its accuracy, and this validation is likely to be specific to different populations. Because a lack of activity may not always indicate sleep, data from other sensors used simultaneously may be needed to increase accuracy. Another discussant suggested that the field may be too focused on getting readouts of sleep stages and that a simpler measure like heart rate may be sufficient. As researchers spend time validating a plethora of commercially available fitness devices, it could be useful to collaborate with companies to produce a device specifically for sleep research.
Three moderators made comments and guided discussion to help process the many explorations of sleep in ASD. Moderator Federico Bolognani from Axial Therapeutics reiterated the need to define an objective when measuring sleep, understand its feasibility in ASD and validate it against other measures. Andrew Varga of Mount Sinai recommended hypothesis-driven and family-centered approaches and encouraged study of the role of circadian rhythms in sleep disturbances in autism. Emmanuel Mignot of Stanford University noted the challenges in choosing among potential sleep measurement devices, though he acknowledged that a measure of activity as a first screen had value. He also emphasized the need for obtaining raw signal data from the device, as well as context-specific validation.
Even as devices proliferate and databases are established, Dijk suggested that the field still lacked a basic characterization of sleep in ASD and that a survey of sleep patterns by objective and by self- or carer-reported measures could help parse some of the heterogeneity found in the disorder. One approach would be to collect massive amounts of data from wearable technologies, then let deep learning of the data find distinct sleep patterns. An alternative approach would be to collect multiple kinds of sleep data from a targeted group, such as those with PMS, though feasibility would be an issue.
In contemplating future clinical trials of sleep interventions, Chris Winrow of Cyclerion stressed the importance of keeping subjective measures of sleep alongside the newer objective measures. This is because regulators want to see that a treatment produces improvements in sleep or quality of life, not just a change in a sleep biomarker. Mignot echoed this, saying that a wearable measure is useless without a clinical feature of sleep alongside it, preferably a validated patient-reported outcome measure, though there may be a need for designing a new tool. Others added that the subjective experience of sleep, and how people feel while awake, could also be useful indicators.
In closing, SFARI’s interim director John Spiro welcomed the community’s continued input about how to use SFARI cohorts to address some of these questions, as well as how SFARI itself could help promote sleep studies in ASD. “Obviously this is just the beginning of a long conversation,” he said.